Cloud Computing: Optimizing IT with Artificial Intelligence
Henrik Hasenkamp from gridscale explains how data analytics in the data center itself can be used to increase performance, reliability & security.
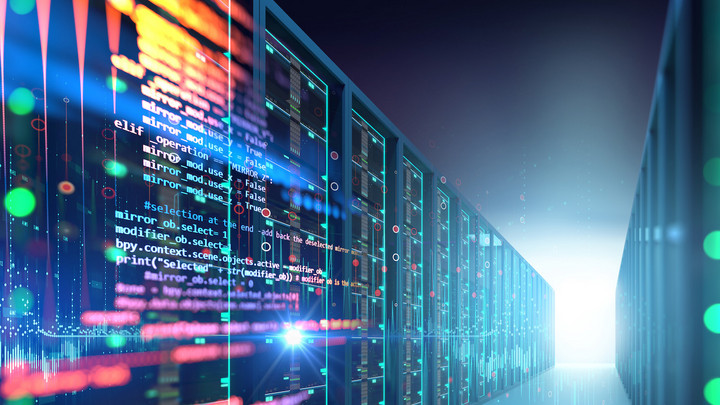
© monsitj | istockphoto.com
One of the main triggers for companies to opt for cloud computing is the high quality of service that comes with such solutions. With the help of artificial intelligence (AI), the cloud can learn to make optimal infrastructure decisions in various situations and environments. The key to efficient use of AI is data. While the data center provides sufficient data information, the real challenge is in interpreting it correctly.
Intelligent data analysis already makes a valuable contribution to business today: sales increases through data-driven up-selling, and cost savings through data-based optimization of the production environment are just two examples. Data analytics can go far beyond preparing a management report at the end of the month. Rather, it is a matter of interpreting data in such a way that it unfolds its full preventative benefits – for example in the data center.
It goes without saying that the online shop of an e-commerce company needs to be reliably open and functioning around the clock. Cloud concepts have proven their worth in order to scale, when required, and, for example, to absorb seasonal peak loads without having to provide expensive resources. So far, so good. But what if the reliability of the infrastructure could be increased even further by predicting certain events, such as additional resource requirements or the declining performance of a website?
Use data that is already existing
Cloud concepts bring flexibility and cost transparency. However, the higher the availability and the faster it can be scaled, the more expensive the package is for the customer. This is by no means unfair, as the provider has to manage and maintain the appropriate resources in order to be able to offer its customers a high level of flexibility and responsiveness. Thanks to AI, it could be possible to manage cloud resources more precisely, more individually, and at lower costs.
This is because the data that the infrastructure components of a data center provide during operation can easily be used to give valuable insights: The moment an online shop is no longer accessible, for example, the operator suffers sizeable damage. It is very likely, though, that long before the website failed there were clear technical signs that problems could arise in the near future. If these had been noticed in time, the failure could have been averted.
Most incidents are preceded by detectable anomalies within data center operations. Before a website crashes, it’s possible that an unusual traffic peak was already visible, the CPU was working at the limit of its capacity, and access requests for the database had spiked. Likewise, even before an attack takes place, hackers leave traces, such as a high number of login attempts or other unusual activities in the network.
Getting such data is comparatively easy, because most hardware devices of the typical IT infrastructure have the necessary sensors built in. This allows a high volume of status data as well as operational data to be recorded, such as the temperature of the devices, latency times, the number of write and read access instances, log files, and the like. The more difficult question is how the data can be put into the right context, so that conclusions drawn from it will be correct. Behind an increased access rate, for instance, could be either a hacker or a run on seasonal goods due to an advertising campaign.
Only a learning system is an intelligent one
The system therefore must first learn what must be defined as an anomaly in a completely neutral sense. To impose a construct from defined situations on the algorithm is of little use, as it is hard to set limits in advance as to which value change has which meaning. Instead, several data measurements, with changing inter-dependency, always play a role.
In concrete terms, this means that the algorithm has to learn. To achieve this aim, a manageable number of features must be defined, i.e. values with their possible characteristics which are important for the operation (such as the above-mentioned example for the operation of the website). The more features that are observed, the more accurate the analysis becomes – but at the same time, the more complex the system becomes. During operation, all events that are remarkable in any way are now marked for the algorithm: planned seasonal load peaks, for example, or unpleasant performance bottlenecks. Over time, the system can interpret situations itself and provide the basis for an intelligent warning system.
Predictive maintenance in the data center
The important top level of such a warning system remains the immediate alarm, if a value stands out so strongly that immediate action must be taken. If, for example, the data stream of a hard disk breaks off abruptly, the disk may be broken.
Reactive maintenance of IT infrastructures is a common model that achieves high availability thanks to security measures such as clusters and redundant data storage. The higher the desired availability, the more expensive it is.
The second level of such a warning system is based on the AI of the learning algorithm. Based on the defined features, the developments of their values, and the trained correlations, the system can now work predictively.
If the recorded values and their changes – within a certain period of time and under defined conditions – indicate an unwanted anomaly, the IT administrator will be automatically informed. The advantage of this is that the manager can decide to intervene immediately or choose a suitable time for the upcoming maintenance.
On a third level, an intelligent system can be developed from a pure warning system into a system which optimizes the infrastructure. Cloud providers, for example, can successively scale resources so that true live-scaling becomes possible, even without user intervention.
Automated infrastructure adjustments are also possible: If a device is permanently running under maximum load, this can be resolved by adding another device before any performance losses occur. The algorithm can decide for itself which is the most practicable, cost-effective, or simply urgently necessary measure for a specific case.
Optimized Infrastructure as a necessary basis for a successful business
An efficient and highly flexible infrastructure is essential in order to remain competitive, particularly in the e-commerce industry. It is not always easy to find an optimal balance of price and performance. A cloud which uses learning algorithms is able to make the necessary decisions depending on the situation, and thus optimize infrastructure operation with the right balance of price and performance.
As the degree of automation increases, the company’s need to put work into this decreases. An AI-based system also has advantages from a security point of view: Ransomware attacks, for example, cause a particularly high read and write rate that a normal user cannot achieve. If the algorithm detects this anomaly, countermeasures can be initiated considerably faster than ever before. Data-based predictive maintenance has been gaining in importance in the industry for some time now. Why not transfer this cost and time-saving concept to data centers?
As CEO of gridscale, Henrik Hasenkamp is responsible for the strategy and development of the European IaaS and PaaS provider, based in Cologne. Even before he co-founded gridscale in 2014, Henrik was firmly rooted in the hosting business. He worked at PlusServer AG, the IaaS provider ProfitBricks, as well as at the Vodafone business division “Cloud & Hosting Germany” and the Host Europe Group.
Please note: The opinions expressed in Industry Insights published by dotmagazine are the author’s own and do not reflect the view of the publisher, eco – Association of the Internet Industry.