AI meets Distributed Ledger: Building Marketplaces, Sharing Data, and Breaking Silos
Artificially intelligent decisions must be trustworthy, both to gain acceptance & acceptability and to advance Europe as a leader in AI, says Prof. Ali Sunyaev from KIT.
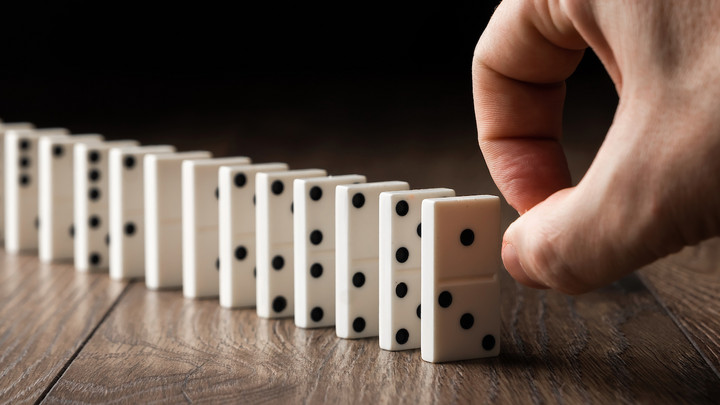
© MARHARYTA MARKO | istockphoto.com
This interview was first published on the Service-Meister website. Reproduced here with kind permission.
Nils Klute: Prof. Sunyaev, why does artificial intelligence (AI) need to be trustworthy?
Prof. Ali Sunyaev: Because trustworthiness makes AI more reliable for all parties involved. Not only to prevent harm, but also to be able to understand decisions and predictions of AI when needed or in certain contexts.
Klute: What does that mean exactly?
Sunyaev: For example, that an AI complies with rules, laws, and regulations, upholds ethical, social, and societal norms, and, of course, is technically robust.
Klute: Why does the industry need such an AI?
Sunyaev: To give you an example: In a factory, AI autonomously controls systems and devices. If humans and machines are working side by side, it must be ensured that an AI is able to distinguish between employees and objects at all times. If real-time processes are intelligently automated, this is not only technologically challenging, but also an issue of safety. When AI is used at the management level, it should be ensured that predictions and recommendations of AI follow ethical and social principles, as must all economic activity.
Klute: What are the key issues?
Sunyaev: Applications of AI are still far too often a black box. How neural networks make predictions is difficult or impossible to understand, even for experts. By the way, in some contexts this is also desirable or even required – in most cases, however, the opposite is the case. Furthermore, AI is by no means a neutral advisor. Every decision is tainted by prejudice, a bias to which the data processed by an algorithm are themselves subject. For reasons like these, IBM announced in early June that it would no longer offer facial recognition systems to the police.
Klute: And what is a possible solution?
Sunyaev: You could make AI more reliable by combining it with Distributed Ledger Technology (DLT)...
Klute: ... so algorithm meets blockchain?
Sunyaev: Not quite, the concept of blockchain also provides for algorithms. Rather: AI applications and DLT systems can be combined.
Klute: What are the advantages?
Sunyaev: For one, AI can detect vulnerabilities in DLT systems. Examples are the detection of software bugs in Smart Contracts or game theoretical analyses of the fairness of DLT systems. On the other hand, DLT systems can be transferred to AI; for example, to document decisions or predictions in a completely traceable manner, to ensure the integrity of training data, to protect access rights, and to manage identities, so that it is always clear what an AI is allowed to do and what not.
Klute: What are applications for this in industry?
Sunyaev: Decentralized data marketplaces can be set up, information can be securely shared, and silos can be broken. For example, Federated Learning allows AI agents to be trained locally where the industry stores data. In the end, companies do not share their raw data, but rather aggregate AI models, whose integrity in turn is ensured by the DLT. In this way, competitive interests are safeguarded. Also, if too little training data is available in a company, the distributed approach solves the dilemma.
Klute: How can this help in the Service-Meister project?
Sunyaev: Used in maintenance, DLT can ensure that training data is trustworthy. Or, if service data is only available in small quantities in decentralized and locally distributed form at user companies, Federated Learning could help to teach AI applications in the first place. Aggregated and mature AI models can be exchanged in a more tamper-proof way, which, for example, enables better predictions for predictive maintenance. A result that benefits all participants in the service ecosystem for Industry 4.0 – without having to disclose their data.
Klute: How well do industrial services and trustworthy AI fit together for you?
Sunyaev: Trustworthy AI from Germany and Europe has the potential to become a successful product. Especially a project such as Service-Meister around the B2B platform market is certainly exciting here – not only to advance intelligent machine maintenance, but also to establish Europe as a leader for reliable AI.
Klute: Thank you for the interview!
Ali Sunyaev is Professor of Computer Science and Director of the Institute of Applied Informatics and Formal Description Methods (AIFB) at the Karlsruhe Institute of Technology (KIT). With more than 9,000 employees and an annual budget of almost 800 million Euro, the KIT is one of the world's largest research and teaching institutions with the potential to become a global leader in selected research fields.
Please note: The opinions expressed in Industry Insights published by dotmagazine are the author’s own and do not reflect the view of the publisher, eco – Association of the Internet Industry.