Trusting in AI: Why Algorithms Have to Explain Themselves
Wherever AI supports people, above all trust is needed in the technology. Nils Klute of the eco Association explains how we can test and certify trusted AI applications.
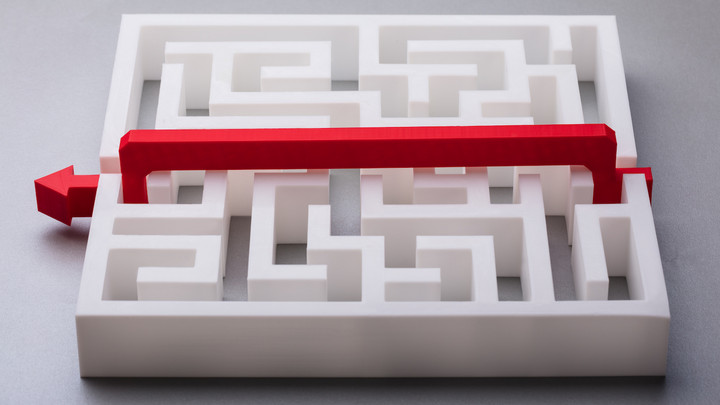
© AndreyPopov | istockphoto.com
This article was first published on the Service-Meister website. Reproduced here with kind permission.
Cars drive autonomously, software diagnoses cancer and machines control themselves – artificial intelligence (AI) makes it all possible. But wherever AI supports people, trust in the technology is needed above all. Here is how we can test and certify trusted AI applications.
AI helps in the fight against cancer. From X-rays to CT scans and MRI images – AI can evaluate them and assist physicians in making a diagnosis. To do this, the algorithms analyze an enormous amount of data faster than is humanly possible; they mark tumors on the images and present the results to the experts to take a decision on. Consequently, physicians can initiate therapies earlier and gain time for their patients. However, this improvement in healing prospects also comes with problems since it is not clear which characteristics AI uses to distinguish dangerous metastases from harmless cysts.
Many AI systems are non-transparent. And not only in the health sector. It turns out that the way neural networks are modeled on the brain and how they are essential for machine learning is a bit like a black box. Even their creators do not fully understand what is going on inside them. People can justify and explain their behavior – neural networks cannot. This is a fact that raises questions in areas where smart algorithms already act largely independently, but where decisions must be comprehensible to humans. As a result – whether it be in the automated granting of micro-loans, personnel selection, or future traffic systems – people are becoming suspicious and fear losing control, as demonstrated by an Audi study on autonomous driving carried out among some 21,000 consumers worldwide.
Evaluate, understand, and explain
The solution is called Explainable Artificial Intelligence: XAI makes AI explicable and makes algorithmic opinion formation comprehensible to humans. But, how can this be achieved in practice? One example is to certify AI systems; something the Fraunhofer Institute for Intelligent Analysis and Information Systems IAIS is working on. To this end, the Bonn-based company has formulated a catalog of requirements for trustworthy AI, which enables intelligent applications to be evaluated expertly and neutrally. The aim here is that certification should guarantee technical reliability and responsible use of the technology. Not only are the AI decisions checked for transparency, they are also checked to see how fairly, securely, and reliably algorithms actually work.
Do AI applications protect sensitive information? How reliably do the applications work? And are AI systems secure against attacks or errors? “The problem with many neural networks is that they cannot be broken down into modules that can be tested separately,” says project leader Dr. Maximilian Poretschkin on heise.de. Instead, the experts examine how AI works by looking at the assured properties of an intelligent application.
Highly dynamic, self-learning, and challenging
What makes this project challenging? Test objectives cannot be standardized because there are currently no specifications or standards for AI. For example, when vehicles undergo safety inspections, the brakes, engine, and lights are tested as standard; however, these kinds of standard inspections do not exist for algorithms. Furthermore, AI applications are highly dynamic. Whether self-optimizing, self-organizing, or self-learning – where software works with versions, AI systems develop autonomously and continuously.
The solution is provided by a framework developed by the Fraunhofer Institute and presented by Poretschkin in a webinar organized by the eco Academy (in German). The audit catalog can also be applied in a structured manner to areas of activity such as fairness, security, privacy, or transparency. The framework looks at the complete life cycle of AI applications. This includes, for example, the design and architecture of the applications, the training and input data, the algorithm, and the behavior of the AI components themselves.
Documenting, checking and testing
How might the certification be conducted in practice? AI developers document their work according to the catalog and auditors assess the plausibility of achieving the objectives. The standardized procedure looks at typical risks, defines KPIs, and includes everything in the overall assessment. “Depending on the criticality of the AI application, a greater depth of testing is also required, which goes beyond a purely documentation-based assessment. We are developing appropriate testing tools for this purpose,” says Poretschkin.
And it’s worth it. After all, only those who trust AI will use it. A current AI study by eco – Association of the Internet Industry shows that if the technology is applied across all industries and to its full extent, a total economic potential of around 488 billion Euro can be released for Germany alone.
Join the discussion on our Service-Meister LinkedIn Group here: https://www.linkedin.com/groups/8912754/
Nils Klute is Project Manager Internet of Things at eco – Association of the Internet Industry. He covers topics such as smart cities, smart factories, and smart homes. Prior to his start at eco in 2018, Nils worked as a corporate journalist for IT corporations (like SAP, T-Systems, and QSC at Cologne-based communication agency Palmer Hargreaves) and previously held public relations positions at market and economic research institutions.