The AI Privacy Dilemma
Can we have advanced efficient Artificial Intelligence while protecting digital privacy? Rainer Sträter from 1&1 IONOS and Dr. Leif-Nissen Lundbæk from Xayn give us their answer.
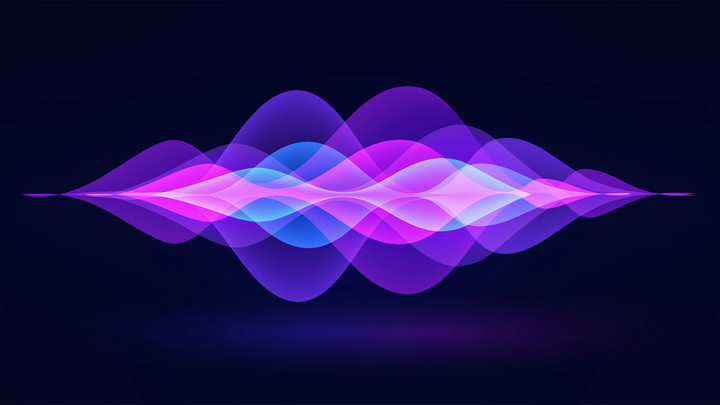
© Chiken Brave | istockphoto.com
"Will AI enslave us all?” or “Will AI save humankind?”. The discussion around AI seems to oscillate only between these two extremes. This is good news for click-baiting but hinders a realistic debate about the real challenges. The situation is far more complex because AI has the potential to be both – or as Sune H. Holm, associate professor of philosophy at the University of Copenhagen, put it: “On the one hand, we can use these systems to prevent serious harms to people and society. On the other hand, using these systems seems to threaten important values such as privacy and autonomy.”
What is the AI Privacy Dilemma?
If we want to tackle this challenge, we need to have objective discussions about the potential problems and solutions. One of the central topics in this debate is the so-called AI Privacy Dilemma, which can be explained as follows: To train AI models, large amounts of data are necessary. The common approach has been to collect as much user data as possible – the more, the merrier. However, the more data is collected in one place, the greater the danger that this data will one day be misused. It might be used for purposes other than the original ones, it might be sold to a third party, the company collecting the data might be bought by another company, etc. And even if collected data is not used or sold for other purposes, there is still the danger that it might be stolen. Just remember when information on all three billion Yahoo accounts was leaked in the potentially biggest data breach in history.
Are there technical solutions to the AI Privacy Dilemma?
So far, the norm has been to collect data from the devices where it was originally created to process it centrally. For privacy reasons some form of data anonymization method – such as differential privacy – might be applied, modifying data slightly before processing it. This is used widely because it is relatively easy to implement.
However, by combining different databases, personal data can be recalculated and while anonymization technologies are improving constantly so are deanonymization technologies. Therefore, this is one of the methods with a lower privacy rating.
To really reconcile AI and privacy, we should question the entire process of collecting and processing data centrally and think about different approaches – e.g., edge computing and especially masked federated learning. Here, the algorithms are brought to the end devices where the data is created. These local models are encrypted and sent to an aggregator, which is the basis for subsequent local learning. All personal raw data stays on device level at all times, only updated models get communicated in the network in an encrypted way. This way, privacy is built into the process right from the start. Masked federated learning combines the best of both worlds: a highly efficient and privacy-protecting AI.
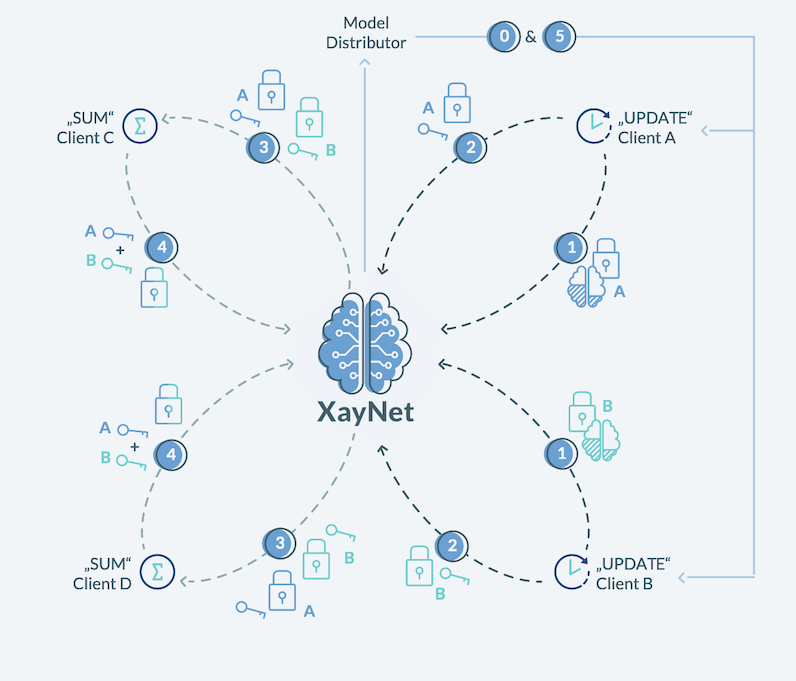
Fig. 1 ©Xayn AG: Our PET solution for federated learning (1) “update” participants send their masked local models to XayNet and their seed of the corresponding mask – via XayNet in (2) and (3) – to “sum” participants. XayNet receives the sum of masks from “sum” participants (4), uses that to compute the aggregation of masked local models, and (0 & 5) sends this new global model to a Model Distributor which passes it on to relevant parties of the use case.
Also, large amounts of data don’t have to be shipped anymore and decentralized systems are more resilient against attacks. Potential attackers would have to focus on an enormous number of different devices at the same time to succeed – a highly unlikely event. Therefore, this decentralized approach can accommodate data-protection directives such as the GDPR with greater ease, scalability, and cost-effectiveness.
One example of an application using masked federated learning is the recently launched European search engine Xayn, which combines personalized search with data privacy. It uses a proxy to hide user information from the various indices, and tailors the results directly on device level to the preferences of the individual users. This way, users receive personalized search results and can actively train the underlying AI models to their needs directly on their device, while their data stays on their devices.
Privacy-proofing a company’s infrastructure
To further privacy-proof their services and tools, companies also should make sure that not only the technology itself but also the underlying infrastructure is compliant with current privacy regulations such as GDPR – e.g., the cloud infrastructure. Even though a common approach is to use one of the global leaders in cloud infrastructure, it might not be the most privacy-conscious one.
The reason: The legal regulations to which US providers are subject (US CLOUD Act) contradict the European idea of data protection and thus the GDPR. The CLOUD Act requires US companies to disclose data stored or processed outside the United States to authorized US authorities without a court order. Companies located in Europe are also subject to the CLOUD Act if they are a part of a US company or exchange data with US organizations.
The Cloud Act requires companies to not only disclose their own data, but also all data in their possession, custody or control, including customer data held by a cloud service provider. It includes both personal and company data – from commercial information to trade secrets to intellectual property.
This way, companies in Europe run the risk of violating either the US Cloud Act or the GDPR. Every European company should take a very critical look at the impact of this regulatory basis. Only cloud providers with headquarters AND data centers in the EU offer maximum protection from the CLOUD Act and are GDPR-compliant. Xayn for example, has chosen to use the cloud platform of the European provider IONOS for its purposes.
Being privacy-proof to become future-proof
In public debates, privacy is sometimes seen as a great obstacle for development in Europe. Some politicians even argue that we should compromise on data protection to enable new technology. Some companies support this claim by stressing that they wouldn’t be able to provide their services without collecting large amounts of data – but that is only part of the truth. In fact, in many cases the services themselves don’t depend on the amount of data collected, but rather the business model of the company.
This is especially relevant as we create more and more data with every minute that passes – and this trend will only continue. The official EU data strategy projects an increase of 530% in the global data volume, up from 33 zettabytes in 2018 to 175 zettabytes in 2025. The same study also suggests that even though in 2018 80% of all data was processed centrally, by 2025, 80% of data will be processed on device level.
In addition, consumers exert more bottom-up pressure on companies to make sure that they protect the data of their customers – as demonstrated recently by the mass exodus from WhatsApp to privacy-protecting Signal. EU lawmakers should seize the moment, because “the EU’s strong emphasis on the more social, ethical, and consumer-friendly direction of AI development is a major asset. But regulation alone cannot be the main strategy. For Europe’s AI ecosystem to thrive, Europeans need to find a way to protect their research base, encourage governments to be early adopters, foster Europe’s startup ecosystem (...), and develop AI technologies as well as leverage their use efficiently”.
Corporations should react to this bottom-up pressure from consumers and the top-down policies by lawmakers proactively. The sooner companies start to privacy-proof their technology and infrastructure, the more future-proof they will become.
Rainer Straeter is Head of Global Platform Hosting at IONOS. In this role he is leading the international IaaS technology division as well as the international technology teams at IONOS’s subsidiaries Fasthosts and Arsys. In 2015, he was responsible for IONOS’s successfully launched next generation cloud platform in EU and US. Since 2019, he has held a seat in several committees and working groups within Gaia-X.
Leif-Nissen Lundbæk (Ph.D.) is Co-Founder and CEO of Xayn and specializes in privacy-preserving AI. He studied Mathematics and Software Engineering in Berlin, Heidelberg, and Oxford. He received his Ph.D. at Imperial College London.
Please note: The opinions expressed in Industry Insights published by dotmagazine are the author’s own and do not reflect the view of the publisher, eco – Association of the Internet Industry.