The Open Source Curriculum for Corporate Training on AI and Digitalization
Dr. Fred Jopp, Hauke Timmermann, Christine Neubauer & Andreas Weiss present an advanced training approach on AI in technical service, developed to support SMEs in closing the skills gap and keeping abreast of digitalization.
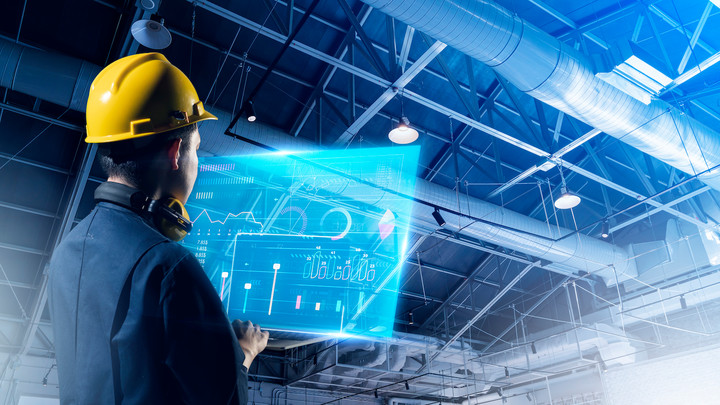
© Thinkhubstudio | istockphoto.com
For some time now, the consideration of total cost of ownership has been shifting away from the pure purchase or production of machines etc. to platform-as-a-service approaches. Here, traditional industries are challenged to develop and expand innovative solutions and solution concepts in order to keep up with international competition. In this context, the structure of technical service is being transformed from the ground up, through the broadening and improvement of the range of services available, while minimizing costs at the same time.
Due to the shortage of skilled workers, human resources do not scale arbitrarily; therefore artificial intelligence is being used to implement new business models, such as 24/7 and predictive maintenance approaches, and to close the gap in human resources. As a result, employees need to be introduced to and trained in the sophisticated new service approaches. Due to the complexity of the procedures involved in artificial intelligence, the previous range of methods must be expanded.
In the following, we present an advanced training approach on AI in technical service, developed as part of the flagship project Service-Meister. Here, we have developed an open-source curriculum for AI in technical service, with which we initially want to address employees from the technical service of SME companies and the skilled trades. In a further step, we will then use a multiplicative train-the-trainer approach to broaden the base, while ensuring an academic connection in a further approach via the universities of applied sciences.
Challenges for SMEs in moving from selling products to selling services
Currently many German companies, specifically SMEs, are facing enormous struggles: troublesome, aggressive, international competition to keep up with, and the necessity of delivering digitalization services successfully by whatever means, even when no digitalization officer is at hand. And finally, the pressure to find skilled workers who can successfully and flexibly deal with the increasingly complex service landscape.
In these service landscapes, an increasingly central building block is that of visioning applications with artificial intelligence (AI) – whose agile and lean understanding, development and maintenance can simply determine the plain success of entire business units, even if these do belong to very well-established units.
This is particularly true because the manufacturing industry is already selling fewer and fewer pure products and more and more services. Accordingly, sales figures are also developing rapidly in this direction, which in turn is leading to an increase in smart service development.
In this setting, knowing how to develop smart services with AI gives companies a head start, and secures competitive advantages. However, this only works if the company's own employees are trained to such an extent that they can follow precisely this path of smart AI tools because they can understand their importance.
Smart servicing and AI tools
There are now suitable smart services for every aspect of corporate life and work; to name just a few categories: 1) technical smart services, such as predictive maintenance, 2) economic smart services as a modern variant of a continuous improvement process (CIP), and 3) sales-related smart services, such as the web store variant of “customers-who-bought-this-hard-drive-also-bought-this-screwdriver!” What all these AI-based tools have in common is that they can participate in the work and creation process, sometimes in a significant way. This basically means that each of these separate AI tools are small software implementation projects which all require buy in by the affected employees.
This means that they must understand the cause-effect relationships underlying the AI’s actions. It shouldn’t be a black box, i.e., it should be clear why exactly an action is being carried out, and what the purpose of this should be. Almost all surveys on software implementation products underline that this is key to user acceptance. It is no longer sufficient to have employees with classic skills in the company; they must also be open and willing to participate in training measures on corresponding digitalization aspects if there is a business need for this. By this a feedback loop can be established to align the activities with the user needs in general.
Knowledge, training & education
How is this situation dealt with from a corporate perspective? This is the subject of regular company surveys: In 2016, for example, around 85 percent of German companies were involved in the further education and training of their own employees. Not only was a very broad mix of methods used to qualify their own employees, quite considerable resources were also devoted to this. This was exemplified by more than 33.5 billion Euro invested in further education measures in 2016 alone (Seyda and Placke, 2017: Die neunte IW-Weiterbildungserhebung—Kosten und Nutzen betrieblicher Weiterbildung, IW-Trends: 44. Jg. Nr. 4, 2017).
The corporate training market is also growing, especially, of course, due to the fact that the demands on employees are constantly increasing as a result of the complex digitalization applications from Industry 4.0, smart servicing, AI tools, etc.
Open-source approach in the Service-Meister project
Since 1 January 2020, we have been working on a major research project called Service-Meister, in which we are tackling all aspects of technical service for Industry 4.0. The goal here is nothing less than the complete digitalization of technical services, starting with the identification of service needs, through automated ticket dispatching and the use of service bots, to the automated writing and analysis of service reports. In all of these service segments, we are developing AI tools in strong use cases together with companies that can autonomously perform these tasks to support service technicians. In 2021, we will generalize our experiences from the use cases here into building blocks in the form of AI templates, and in 2022, we will use this experience to develop an AI-based, cross-plant, cross-department, and cross-company service platform for German SMEs.
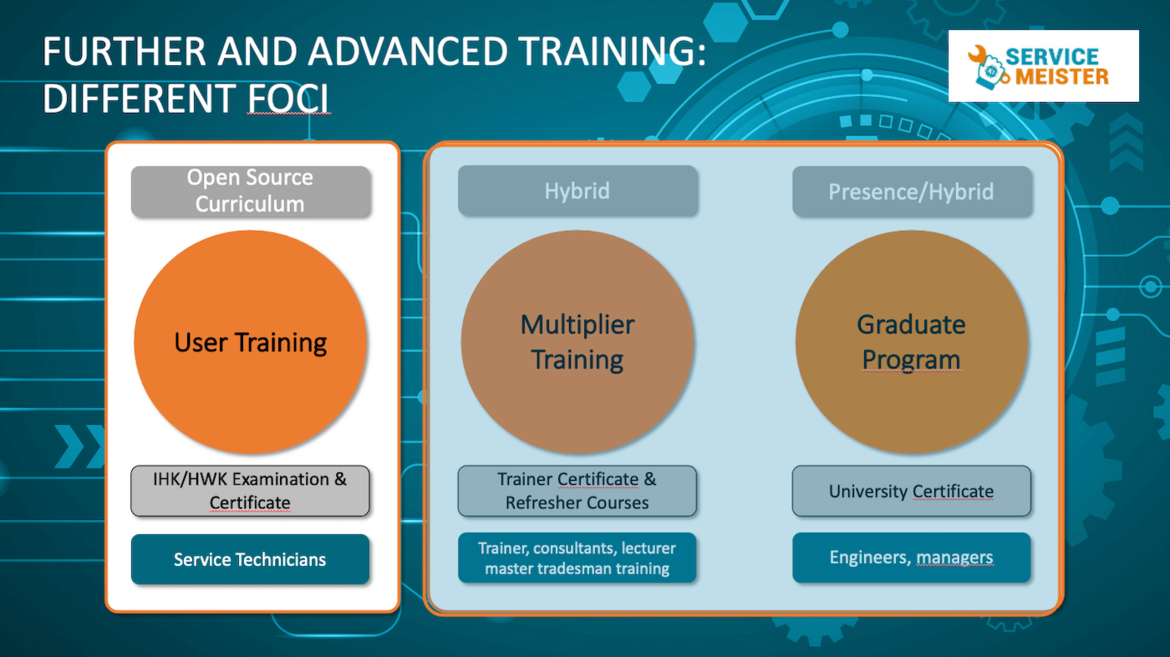
Fig. 1: General structure of the different corporate learning items, from left to right: Open Source Curriculum, Multiplier Training, Graduate Program.
We will then provide a complete service ecosystem that will enable cross-company scalability for Service 4.0. An important sub-goal in this context is to enable less-qualified staff to use and offer complex digital AI services and tools with the help of digital guides. To be able to do this, we have developed an open-source AI curriculum, where we are aiming at:
- Sensitizing SMEs to the technological and economic potential of AI technology,
- Disseminating concrete application examples that are particularly suitable for SMEs,
- Optimally integrating the Service-Meister curriculum via three educational paths with different levels of complexity into existing structures and into the national AI strategy for a transfer of AI knowledge, and
- Creating low-threshold access to AI knowledge for small and medium-sized enterprises.
The target groups for our open-source curriculum are:
- End users / users from companies and the trades,
- Service technicians / service employees from companies and the trades,
- Company employees who are in charge of technical tasks and applications from the content areas of AI, ML and Industry 4.0,
- Participants in training courses offered by the participating Chambers of Trade and Commerce on the above topics; a certificate course is to be created here.
In this open-source curriculum, we provide learning nuggets which cover the following topics:
- AI, Big Data and Smart Data in the industrial environment
- Industry 4.0, Smart Factories, Service 4.0
- Digital business models in industrial environments
- IoT platforms: overview and focus on industry and trade value
- Human-machine collaboration in industrial service
- What is Data Science and how is it applied?
- Machine learning and autonomous systems
- Smart services: technical, economic, sales and marketing
- Examples: Anomaly detection and predictive maintenance
- Examples: Condition monitoring and machine fingerprinting
- What are smart products and how are they created?
- Digital ecosystems
- Service-Meister case studies on the six different industrial use cases.
These topics are bundled together in five learning buckets:
- A practically-oriented introduction to the topic,
- Understanding digitalization,
- When talking about digital transformation, what exactly are we facing?
- With focusing on the most in-demand professions, as represented by the Chamber of Commerce, how can new business models be developed?
- Presenting in detail the six industry-related use cases from the Service-Meister project.
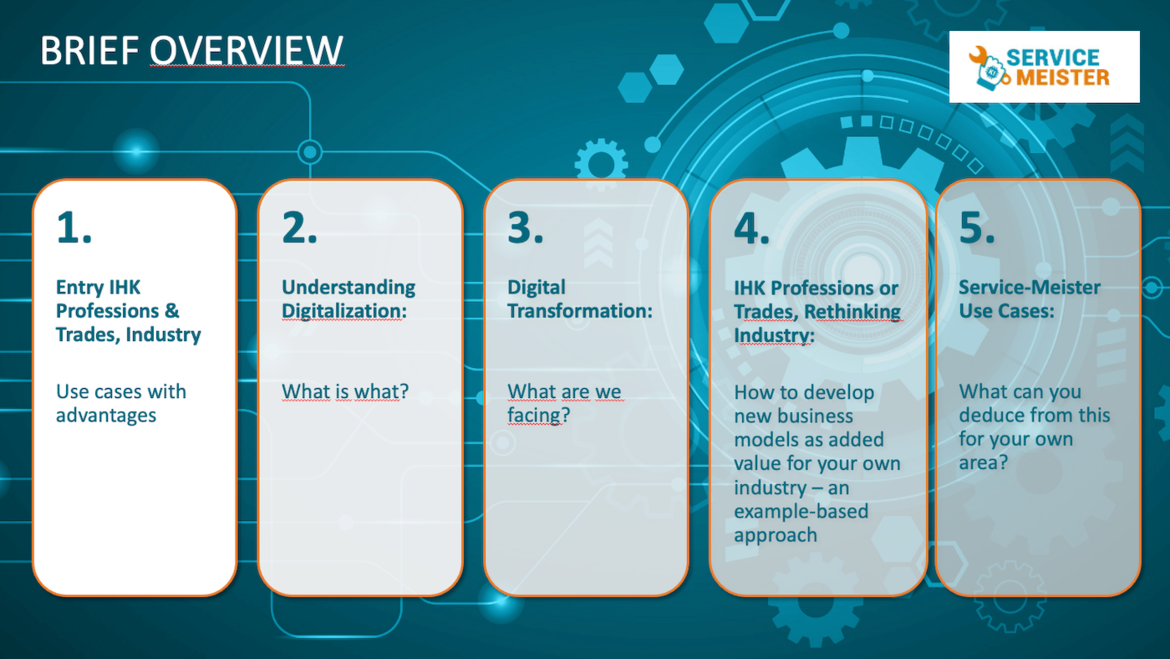
Fig. 2: Brief overview of the learning nuggets within the open source curriculum.
The items from the bulleted list above are assigned to these five learning buckets; for better understanding, we would like to explain in more detail the contents of learning buckets two and three. In the second learning bucket, Understanding Digitalization, the following items are covered:
- Applications of digitalization and AI in the enterprise and why,
- Internet functionality and review,
- Cloud and platform computing,
- IoT platforms,
- How mobile devices, apps, messengers, and social media work, and what the added value is,
- How digital data transfers (W-LAN, xG, Bluetooth) work,
- How service and chat bots work,
- How robotics works and what are the areas of application, and
- A gentle introduction into AI and machine learning.
In the third learning bucket, Digital Transformation, we deal with the following topics:
- An introduction to digital transformation processes,
- Innovation, understanding transformation and disruption processes,
- Addressing actors and enablers,
- Exploitation potential and value networks,
- Opportunity and risk,
as well as a few hot topics:
- Addressing skills shortages through AI,
- Digital office for all,
- Infrastructure outside centers and metropolitan areas,
- How to be legally compliant using data.
The following fourth block on how to develop new business models is an adapted easy dive-in for understanding how to learn to transfer classic approaches from the business canvas to your own professional situation. After a simple introduction to the wide world of digital business models, a world-cafe approach is used here to enable a transfer of the principles of model development to the participant’s own situations and the overall results are presented together. The goal here is to enable an autonomous approach also for representatives who come into contact with the idea of a new business model development for the first time in connection with this learning bucket.
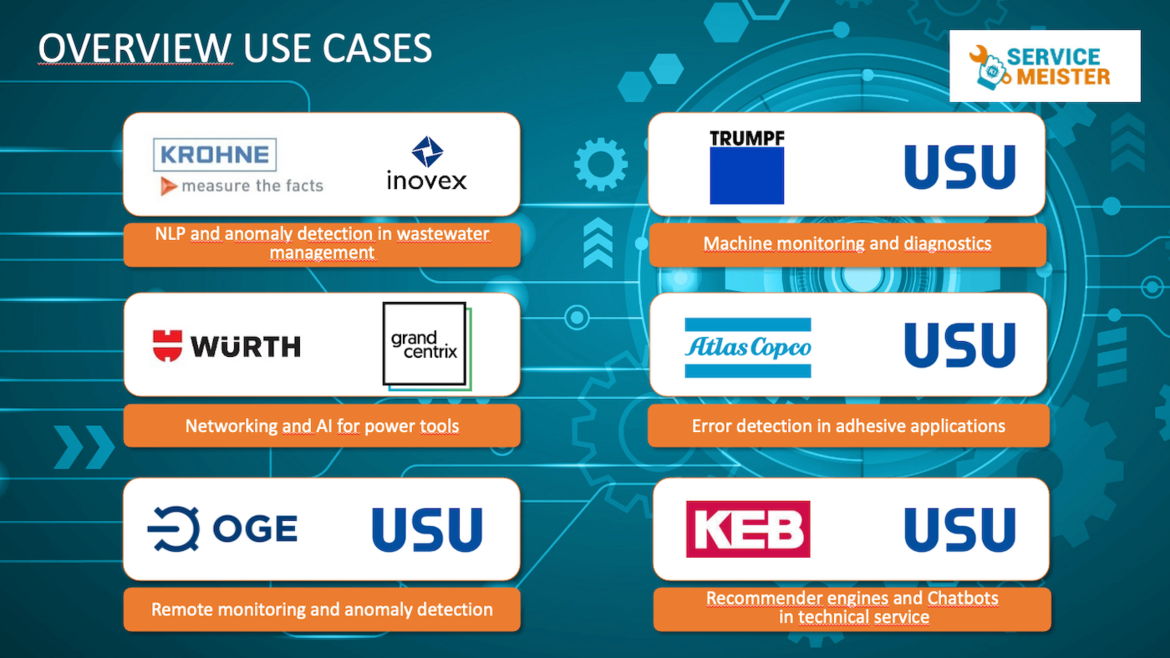
Fig. 3: AI-based Industrial Use Cases of the Project Service-Meister.
In the fifth learning bucket, we present in detail the six different industrial use cases from Service-Meister. The following topics are dealt with intensively, focused on practical experience:
- Anomaly detection for wastewater management,
- Machine monitoring and diagnostics,
- Networking and AI for power tools,
- Error detection in adhesive applications,
- Remote monitoring and anomaly detection, and
- Recommender engines and chatbots in technical service.
Pedagogical approach and outlook
AI and its applications must be made transparent and comprehensible; black-box approaches are not target-oriented. This is why we organized the curriculum into small learning units, the so-called learning buckets. The basic goal here is to build a simple, comprehensible structure, the value of which could also be developed in the process of supported self-learning or digital learning without face-to-face teaching, if necessary. We are also investigating e-learning tools which are suited to our content and approach.
If these goals are considered in further funding rounds for Service-Meister, these could be implemented in the user guidance, with explanations provided through professional video sequences, animations and explanations, micro gaming, the use of topic-related quizzes, and gamification.
But first, we are looking forward to the implementation of the open-source curriculum, which we are planning for the end of March 2021. When this has been completed, we will make the content completely free and accessible to support corporate training. Making something completely comprehensible and transparent not only makes it easy to integrate it into everyday work, but also makes it controllable, thus combatting the feelings of anxiety that many still have in connection with AI.
Dr. Fred Jopp has more than 20 years of experience in Big Data analytics and algorithm development in Applied Physics and Economics. As Head of Industrial Project Management at USU, he is responsible for the development and management of industrial projects in the field of Smart Services.
Christine Neubauer is Project Manager for AI and Industry 4.0 at eco – Association of the Internet Industry. Currently, she is working on the Service-Meister project, an AI-based Service Ecosystem for Technical Service in the Age of Industry 4.0. She is part of the project coordination team, with distributed tasks such as planning, steering, agile actions, reactions, internal and external communication, and stakeholder management.
Hauke Timmermann is Consultant for Digital Business Models at the eco Association and is the contact person at eco for the KI.ASSIST project.
Andreas Weiss is Head of Digital Business Models at eco - Association of the Internet Industry. He started with eco in 1998 with the Competence Group E-Commerce and Logistics, moving afterwards to E-Business. Since 2010, he has been leading the eco Cloud Initiative as Director of EuroCloud Deutschland_eco and is engaged in several projects and initiatives for the use of artificial intelligence, data privacy, GDPR conformity, and overall security and compliance of digital services.
Please note: The opinions expressed in Industry Insights published by dotmagazine are the author’s own and do not reflect the view of the publisher, eco – Association of the Internet Industry.