Cancel Culture for Artificial Intelligence or Just Handcuffs?
If AI systems are not transparent and their benefits are unclear, trust falls by the wayside, writes Dr. Fred Jopp from USU Software AG.
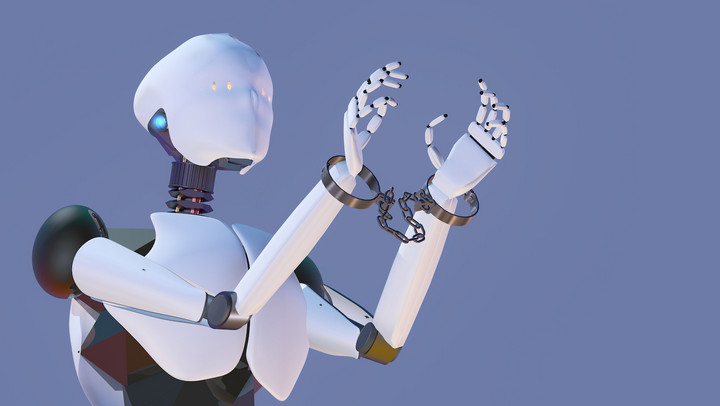
© AYDINOZON | istockphoto.com
How to build necessary trust in AI and how to put the technology into practice. Entrepreneurs are certain: AI is changing the business world – but not their own company, as studies show. A fallacy that has many causes. If AI systems are not transparent and their benefits are unclear, trust falls by the wayside.
It seems like everyone uses the metaphor of oil for the machines of the 21st century whenever we talk about AI, algorithms, and Big Data. What macroeconomic implications are we talking about when we look at this metaphor from a business perspective?
It is the image of the oil pump constantly moving up and down as a symbol of long-lasting, exploratory wealth, in which entrepreneurial characters like Henry Ford I were dominant. He was the first to truly optimize industrial manufacturing, which culminated in the development of the production line for the Thin Lizzy, a car known as the T-model. In the subsequent distribution of the new vehicle, oil prices rose rapidly. Coal shortages, the global economic crisis, and wars made oil a highly strategic resource. Having it at one’s fingertips has decided nothing less than the rise and fall of companies, corporations, and countries.
Meadows (1972) certainly gives this picture a more sustainable, contemporary interpretation. However, the basic entrepreneurial idea at the time with regard to the strategic resource of crude oil was certainly linked more to a focus on opportunities than to the contemplation of risks.
Don’t think in steel, instead benefit from AI
What does this mean for us today and the use of AI in Germany, the country of mechanical engineers? All figures clearly indicate that AI is also such a strategic resource (as the Paice study from 2018 or the AI study of the eco Association from the year 2020 show).
The presumed economic added value that AI will generate for us over the next five years will be in the region of around 50 billion Euro. A crucial contribution that has already brought about enormous changes. This means in concrete terms: Industrial value creation is based less and less on machines, but more and more on AI-based services built around machines. Wilfried Schumacher-Wirges (IoT Solutions, KEB Automation, formerly Heidelberger Druckmaschinen), one of the pioneers of digital service in the German mechanical and plant engineering industry, summed this up succinctly with the remark: “Those who think exclusively in terms of steel today are missing the opportunity to earn money with services!”
For precisely this reason, the examination of the digitalization of technical service via AI tools is the central approach of Service-Meister. The AI flagship project will initially develop AI tools for the segments of the technical service sector which are planned as the foundation for a reference architecture. The goal: When the project ends in 2022, a service ecosystem that spans plants, departments, and companies should be available to German SMEs. In doing so, Service-Meister aims to both give companies with AI a competitive edge in the service field and to counteract the pressing problem of a shortage of skilled workers. The AI project makes maintenance know-how scalable and helps medium and small companies to put AI into practice so that they too can benefit from it.
Few use AI, many fail to recognize the opportunities for their own company
If the digital world around us, as the center of the mechanical and plant engineering industry, is clicking away ever faster, then the expectation should be that the corporate view should increasingly focus on the profitable possibilities of AI, i.e., the creation of more economic value added through the use and exploration of AI tools within each company. And all this in the spirit of exploring a strategic resource, as Henry Ford I did with the T-Model. Interestingly enough, this does not seem to be the case:
A recent study from the German Economic Institute (IW Köln) in Cologne surveyed 686 companies from industry and industry-related services on the status quo of their own AI use. One out of ten companies
is already using AI. At first glance, this reveals an interesting contradiction: When asked about their assessment of the importance of AI in corporate deployment, respondents indicated that AI is potentially seen as a risk rather than an opportunity for their own company and industry. This is especially true for companies that were initially more AI-averse. The majority of the companies surveyed assumed rather globally, on the other hand, that the opportunities were related to the Germany-wide or worldwide significance of AI.
Success of artificial intelligence requires human acceptance in advance
At this point, it is certainly not too daring to draw the conclusion that the use of AI tools has not yet achieved widespread acceptance. This would be necessary, however, in order to compensate for the informational and technological lead of other countries (such as the USA). This is also the conclusion drawn by the IW Cologne study, which identifies open legal questions on intellectual property, liability, and the lack of technical and ethical standards.
All in all, it is important to emphasize at this point that digital transformation is much more than the introduction of a pure technology stack. Rather, the essential moment relates to the mindset and culture in the implementing organization, as illustrated once again by the example in the figure below. This means that the introduction of AI tools is subject to the same mechanisms that apply to software implementation projects. The critical moment here is the acceptance by the members of the organization in which the software entities are introduced.
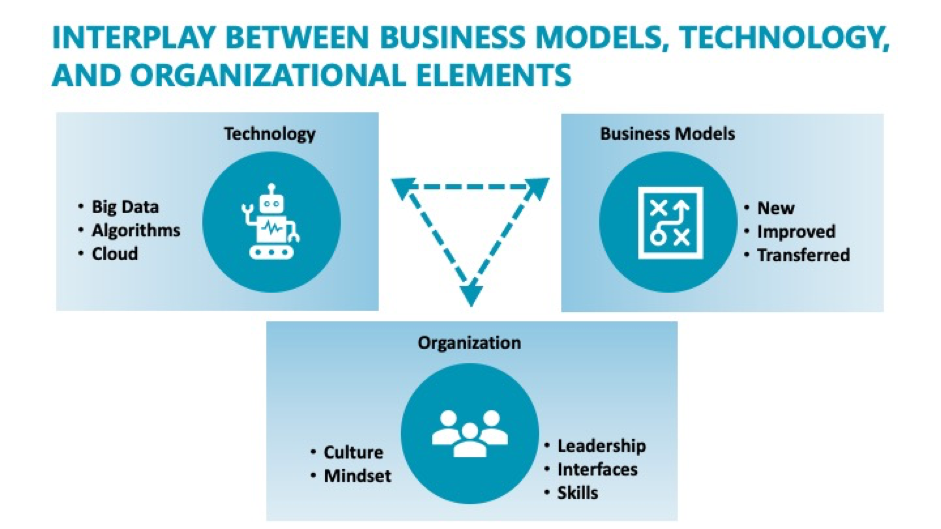
Digital transformation as a field of tension between technology, business models, and organizational elements.
If there is no acceptance or only in part, this has a direct effect on the success rate of the software implementation. The reasons for this are many and varied, such as:
- Establishment of key users who accompany the implementation
- Preliminary surveys, which go in the direction of requirements analyses
- Recognizable benefit of the software for the user
- A successful UX design with intuitive workflow steps
The benefits of AI must be transparent and comprehensible
A central aspect is certainly the point of transparency and traceability of what the software is supposed to do in the operational or business process.
If this is not the case, the benefit can only be accepted a priori, so to speak, or accepted in a result-oriented way, or the result can only be believed.
In this context, the circle with artificial intelligence closes again: So-called black-box modeling is often used here (Jopp et al. 2009). Originating from cybernetics, these are processes in which an external signal is processed as input in a box, and only the result, the output, can be perceived. The actual processing, meanwhile, remains in the dark in the box.
To illustrate this, let’s imagine the following realistic situation from the machine and plant engineering sector: An AI algorithm from the field of predictive maintenance predicts a probability of failure of over 95 percent for an aggregate of a certain machine tool – and this at a point in time that is still several months in the future. What is the background? The algorithm, a Gauss process regression, has learned from the available data of these and comparable machines under which patterns or combinatorics failures are highly likely to occur. The forecast for the machine tool in the example is also based on a fit of the data. How can this assertion be verified for a new operator of the machine? In the “real world”, the safest way is to let the machine “run dry”, i.e. to simply check whether the unscheduled maintenance case actually occurs. And that is a problem: There would be further possibilities for the experienced data scientist to estimate the prediction quality on the basis of the quality of the fit performed by the algorithm. The user in turn can simply believe the prediction or empirically observe whether the case occurs.
Gain and strengthen trust in AI
This realistic AI application example from the mechanical and plant engineering sector shows that black box models pose a major problem in terms of transparency and acceptance. To pick up where the IW Cologne study left off:
Certainly, no confidence is generated by a procedure whose prognosis users can either believe or not. Certainly, the opposite must be the case here. The group of those whom the IW Cologne names in its study as those who consider AI to be worthy of support and implementation – but not in their own company, please – will be strengthened. Corporate policy decisions should certainly (analogous to all political decisions in the public sphere) be understandable in the sense of an evidence-based approach. Otherwise, the much-cited “Cancel Culture” will only get a boost.
Therefore, for the reasons mentioned above, the AI flagship project Service-Meister focuses on the two aspects of AI: acceptance and transparency.
Methodologically, this means that several large work packages in the project deal with the aspect of so-called explainable AI. The aim is to leave the black box approach behind and develop comprehensible quality criteria for the use of AI. Overall, the approach in the overall project is multi-layered, starting with the establishment of standardized protocols for data collection, generation, and formats, the development of accepted AI diagnostic tools, the identification of technician best practices for service requirements, and data management that can be compliant with the respective data governance of the companies.
For these purposes, Service-Meister organizes events with third parties, informs about the current project status, and obtains help and advice from experts in addition to the regular internal meetings on content. “Acceptance of AI: Rejection or trust”, “How does AI arrive among SMEs”, “The future of manufacturing with AI” are just a few of the event titles with which Service-Meister contributes to the dialogue on the acceptance of AI. In order to remain with the image of Cancel Culture, this would mean that AI would not be canceled, but rather expanded, and that flagship projects, such as Service-Meister, would ensure that the handcuffs are taken off AI, especially when it comes to the issue of acceptance and transparency of AI.
Dr. Fred Jopp has more than 20 years of experience in Big Data analytics and algorithm development in Applied Physics and Economics. As Head of Industrial Project Management at USU, he is responsible for the development and management of industrial projects in the field of Smart Services.
Please note: The opinions expressed in Industry Insights published by dotmagazine are the author’s own and do not reflect the view of the publisher, eco – Association of the Internet Industry.