Applying and Perfecting AI Applications for Industrial Use Cases
Henrik Oppermann from USU on AI in the mechanical service industry, companies sharing data and cooperating, and how AI can support humans without replacing them.
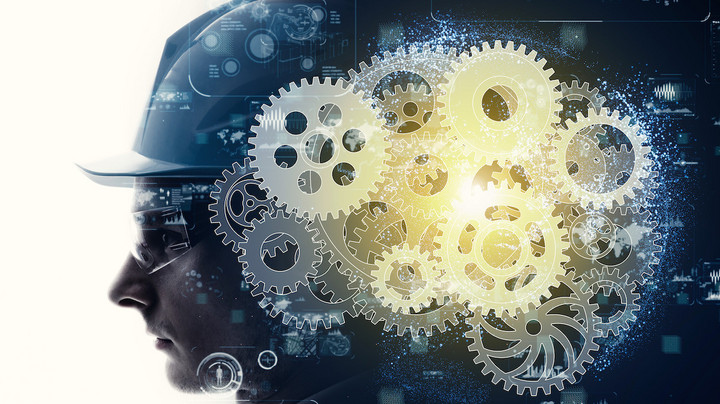
© metamorworks | istockphoto.com
USU Software leads the consortium, which includes the eco Association, involved in the Service-Meister project, developing AI applications in support of the industrial service industry.
dotmagazine: What is the challenge to getting sufficient data for AI training?
HENRIK OPPERMANN: Well, that depends on the AI algorithm or the application itself. Some of them don't even need training. For them it's pretty simple – you won't need any data for the learning corpus.
Then, if we look at the analysis of numerical data, getting this is rarely a problem because machine data is readily available in large volumes. The problem is more about how to handle the mass of data rather than getting it.
For text analysis based on deep learning, this might be different, because to achieve good quality you need a reasonable number of documents. In certain applications, that can be thousands of documents. And I doubt that smaller companies have access to this amount or have manpower to provide them. So, in that case, you would have to apply different algorithms that might help the situation.
dot: How can a win/win situation for sharing data across companies or industries be established? Will companies really share their own proprietary knowledge and expertise with competitors in order to create a cross-industry service ecosystem?
OPPERMANN: I think so, yes. We see that in different domains already. If you're not able to differentiate yourself from the competitor, this might be difficult. So when it comes to direct competition in very tight markets, I think there you may not see much cooperation. But that doesn't necessarily have to be so in the other domains. For example, if you look at the automotive industry, then companies like BMW and Mercedes are sharing efforts to reduce costs for things like autonomous driving or platform development. That helps to achieve goals. And you're still able to differentiate from each other through marketing, and positioning, and such things.
So, in fact, cooperation in very proprietary knowledge-based industries like the automotive industry is already being done. What’s more, in most cases you have a very segmented industry. For example, the printing machine industry is very segmented. They differentiate in certain aspects and address different submarkets. Here, cooperation is already considered, with larger companies with established global service infrastructures conducting services for smaller companies without such capabilities.
Further down the line, lack of qualified employees and increasing product complexity might force companies to intensify cooperation. The latest Lünendonk-List [1] clearly underlined the fact that external services will play a major role in industrial service organizations due to the aforementioned reasons.
dot: Are you seeing that only in Germany, or are you seeing that internationally as well?
OPPERMANN: We are seeing it internationally as well. I think Germans are more reluctant to share, because there is a tendency to think that we have very unique intellectual property, and this makes sharing it more difficult. But, as I said, the larger automotive companies are doing it, and I think this is a global phenomenon. You can see it everywhere.
OPPERMANN: For the technical service industry we are addressing with the Service Meister project, the technical services are international in nature. A lot of companies like Heidelberger Druckmaschinen are operating worldwide, but not in all countries. This means that if one is operating in a certain minor country, and the other one is not, then it's possible to cooperate. You conduct service for your competitor, who in contrast is operating the service for you in another country. So their cooperation is a clear win/win situation as well. And that is something we are addressing in Service Meister. We want to help companies exchange their digitalized knowledge so that they can cooperate better.
dot: How can Europe ensure digital sovereignty in the area of AI?
OPPERMANN: I think that the European Union is addressing a lot of that already, and I'm not sure if more actions are really needed. I think the Europeans - at least I can speak for the Germans - are very good in the application of things like artificial intelligence. We are very good in terms of analyzing machine data and generating smart services for the machinery industry.
As a result, I'm not sure if governmental protections are important. We should concentrate on the application of AI in our domains of strength, i.e. mechanical engineering and automotive. I'm not a fan of closing borders and having our own ecosystem in Europe. I think that would be counter-productive. But of course, we need to define clear guidelines and rules that reflect our cultural and economic background.
I'm not pessimistic about digital sovereignty in Europe. I think we have pretty excellent scientists and very good engineers, and we will be able to compete. There are some rumors that we are way behind in the application of deep learning, but I'm not sure about this. Maybe we are a bit behind in robotics or things like that, but even there I doubt it. I think in the application of artificial intelligence in typical European domains, we are pretty good.
dot: When you say in the application of artificial intelligence, are you suggesting that we're good at making use of AI, but not so good at developing it in the first place?
OPPERMANN: Yes I'd say so. The main improvements in pure technology like deep learning etc. typically come from the American market, and we see a lot of improvements and new developments in the Asian market as well, especially from China. But the algorithm itself is not the most important thing. It's the use case and how you apply it to add value. And I think we are pretty good in applying base technology developed in other markets or countries.
dot: Applying and perfecting, perhaps?
OPPERMANN: Yes absolutely. I mean that's what you have to do. You cannot use one AI algorithm and apply it to all other countries or even all other companies. What we do with our data analysis algorithms is that we adapt them for each customer, because each customer has its own subtleties, its own specificality. And you have to adapt this algorithm to their use cases and to their data.
Smart services are the translation of technology into something that produces value. You have to have a good understanding of the industry, of the domain and their use cases to do this. And we are pretty good at translating base technology into use cases, so called smart services.
dot: The Service Meister project is focused on developing an AI solution tailored for the German industry. Will it be applicable across borders?
OPPERMANN: I think it has to by nature be applicable across borders. As I have already said, the technical service domain is typically international. So most customers we have and most customers we are addressing – initially in Germany – are operating globally. Some of them – even if it's just a one-thousand-employee company – are global market leaders in a certain sub-segment of products, and they are operating worldwide. So the entire idea of Service Meister has to be international for global cooperation.
dot: AI solutions are touted as being a way of addressing the current skills shortage in small and medium sized companies. How fast can viable solutions be developed to deal with the skills shortfall?
OPPERMANN: That's not an easy topic, because that has mainly to do with usability and translating, let’s say, a very complex algorithm into something usable. We have done that partially with our own solutions already, where we hide the complexity of AI from the end user. This can be achieved through a simplified user interface, for example. A second approach is you give end users best practices, some patterns they can use – if they recognize a certain use case, they must be able to match it to something they have done already. That's the main topic: it's more a user interface problem than trying to make the user learn to program AI algorithms.
So what we try to do is bring the skills of the small and medium enterprise into the application. What does that mean? We try to derive algorithms that they can most simply adapt by parameters, or things like that. This can be handled by SMEs as well.
And how fast can such solutions be developed? Well, we haven't done that for deep learning. We have done that for different AI algorithms already, but for deep learning the problem is a little bit different. Most deep learning algorithms operate like black boxes. You put something in, you get results. And this lack of transparency is something that is hardly acceptable for engineers. They have to have the control over the outcome. They have to know why a certain result has been generated by the algorithms. Currently, we have research projects that try to make deep learning explainable and more transparent than they are right now. That's another example of where Europe is pretty good. We know the shortcomings of certain approaches, and we try to make it more usable in domains like mechanical engineering.
dot: As AI starts to dominate the service sector for industry, for example, will there still be space for people?
OPPERMANN: Absolutely. People are still required. For the next five decades, we won't see autonomous robots that you put into service and that can do everything. They can do certain tasks pretty well, but to operate like human beings, with all the experience of humans - we are far from achieving that. Currently, it's not foreseeable. The AIs will have assistant jobs, but they won't be able to conduct complex services autonomously. They will support human beings, and I cannot see that in the coming decades we will have service robots or technicians that are based on artificial intelligence and that can replace human beings entirely.
dot: Finally, what do you expect to be the most promising AI developments in the next five years?
OPPERMANN: I think deep learning and where it will lead us to is very promising. Deep learning is a very powerful approach in artificial intelligence. We have pretty good examples where AI-based algorithms or solutions are better than human beings – for example in the recognition of pictures. Even in quality control, optical quality control in production: such algorithms are extremely good. I'm curious about what will happen with deep learning in the mechanical engineering domain within the next five years. I think that we could see enormous improvements in online production control that will be responsible for much more fault detection and error recognition than we have today.
Henrik Oppermann is Business Unit Manager at USU Software AG and is head of research for the entire group. He is responsible for innovations in the field of machine learning and artificial intelligence and has conducted numerous scientific projects for the company. Henrik graduated in mechanical engineering with a focus on process industry at the Technical University Munich and studied industrial engineering and economics at Karlsruhe Institute of Technology (KIT) afterwards. During that time, he co-founded a startup for knowledge-based systems and, among other things, led industrial research activities for 13 years. He is author of numerous publications in the field of Industrial Big Data, Smart Data, and knowledge-based systems. Since 2012, Henrik Oppermann works for USU Software AG.
Please note: The opinions expressed in Industry Insights published by dotmagazine are the author’s own and do not reflect the view of the publisher, eco – Association of the Internet Industry.