Machine Learning for Small Businesses
ImagineOn provides an overview of how to train artificial intelligence on a budget.
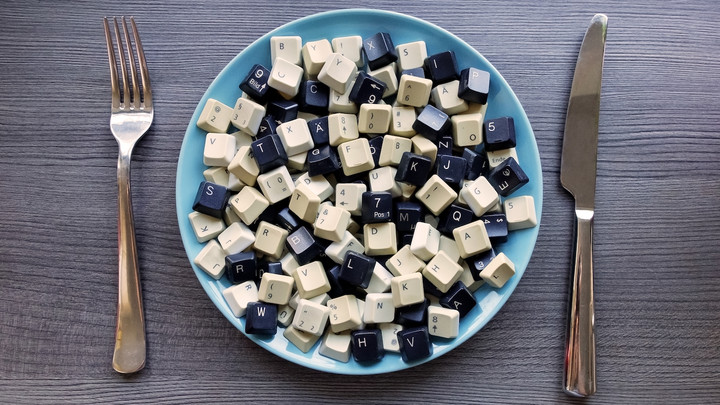
© tookitook | istockphoto.com
This is a shortened version of an article originally published by Dr. Tomás Silveira-Salles in June of 2019 at https://www.imagineon.de/en/insight/machine-learning-on-a-budget
Technology, commerce, science, medicine: machine learning is becoming a part of almost every aspect of our lives and everybody wants to profit from it.
But if you own a small business and want to board this train without having the time or the resources available to your competition, you’ll eventually come across a daunting challenge: collecting data. On the plus side: Not only is implementing a neural network today easier than ever before, but also testing it and tuning it for maximum accuracy is too.
However, to train the network you’ll need labeled data, which consists of examples of things the program should analyze, paired with information it should ideally conclude from them.
For example, if you want your program to analyze images and tell whether they are pictures of cats or not, you could provide lots of pictures of cats paired with the label “cat”, and lots of pictures of dogs and cars and flowers etc. paired with the label “no cat”.
What’s so hard about labeling data?
The sheer amount of data is usually already a problem. Depending on the difficulty of the task and the accuracy that your product needs, you may need hundreds of thousands of labeled examples. It might already be unfeasible for a small business to even get the example data points. Labeling them is then the second part of the challenge.
If you’re lucky, labeling each individual example is an easy task and you can get lots and lots of people to help you. Google's reCAPTCHA is an example of massive online collaboration to create labeled data, in part for machine learning, but also for digitizing books and more.
Do you need labeled data at all?
When we talk about labeled data, we’re actually focusing on an area of machine learning called supervised learning. It is called “supervised” because, during training, the labels tell the machine (for each particular example) exactly what the output should be. This is not, however, the only type of machine learning algorithm available, and in many cases not the most suitable.
For example, when trying to extract the alphabet of an unknown foreign language from a collection of texts written in that language, there is simply no starting point for creating labeled data.
What you would do in this context is scan the texts and use some simple image recognition to extract every single character as a separate image, thus creating a huge set of data points (but no labels). Then, using unsupervised learning, a computer would organize the pictures of isolated characters into groups of pictures that seem to follow the same patterns and therefore probably represent the same character. In the end, each resulting group corresponds to a letter in the unknown alphabet. By giving each detected letter a name you would create a huge dataset of labeled data that could then be used to do supervised learning on new texts to create lists of all words or sentences, etc. in those texts.
Therefore, unsupervised learning is about finding common patterns in the data, without having a predefined list of patterns to look for. Whereas labeling isn’t the starting point, it is nonetheless the aim of the unsupervised learning.
Another approach, called reinforcement learning, is about letting the machine come up with its own guesses (solutions) for each input and telling it how good each guess was, even though no specific solution is expected.
For example, if you want to create an autonomously flying drone, you couldn’t start by telling it exactly what to do with each propeller, but instead let it guess what to do, and then check whether the drone e.g. comes closer to the ground as a result. If it does, the chosen reaction was a bad idea, and the computer should learn from it so this doesn’t happen again. This “evaluation” of solutions can be done automatically (using the drone’s altitude sensor), so no human input is necessary during the training phase. In such a case, labeled data is not a requirement.
But even if none of this helps and you really do need labeled data to solve your problem, hope is not lost!
There are three methods that can be seen as advanced variations of supervised learning and can help you get better results, even when data is scarce.
#1 Semi-supervised learning
Imagine: you’ve decided to learn karate and have signed up for classes. During class, your teacher tells you how to kick and punch and block and jump. Your teacher shows you the moves, guides your body while you’re trying, and gives you feedback after each attempt. After class you practice at home in front of a mirror, correcting yourself based on what your teacher has taught you so far.
Training with your teacher’s help corresponds to using labeled data (appropriately called “supervised” training), while training alone corresponds to using unlabeled data (appropriately called “unsupervised” training).
Machine learning algorithms can use a similar “mixed” approach, called semi-supervised learning.
In semi-supervised learning, a small set of labeled data can be used for the initial training, and a much larger set of unlabeled data can be used to improve the machine’s accuracy or confidence. This can be extremely useful in cases where labeling data is difficult, but there is abundant unlabeled data.
#2 Active learning
Imagine that you have an exam next week. Today is your last class before the exam, and you go to see the teacher in her office to ask a few questions you still have. You know where your weak spots are and so you focus on asking the teacher very specific questions.
Why should machines learn differently?
In terms of machine learning this approach is called active learning. Basically, after some supervised learning with a small amount of labeled data, the machine can analyze its weaknesses and ask you to label a few more (carefully chosen) data points which will help it learn as much as possible.
There are several criteria the machine may use to decide which unlabeled data points would be the “most helpful”. Common ones are reducing the error rate, reducing uncertainty, and reducing the solution set.
The first one – reducing the error rate – is fairly obvious as a general concept (even though the precise meaning of “error rate” can be very complicated).
Reducing uncertainty is less obvious. In most common machine learning algorithms with discrete output – such as classifying pictures as “cat” or “no cat” – the algorithm first computes a value that can be in-between, for example “45% cat and 55% no cat”, and then returns the closest valid output (in this case “no cat”). However, the fact that 45% and 55% are so close shows that the machine is somewhat uncertain about the output, and such cases are the ones the machine is most likely to get wrong. Reducing the uncertainty means training the machine further so that this intermediate value separates the possible outputs more clearly, such as “5% cat and 95% no cat”.
Finally, reducing the solution set is the least intuitive approach.
Imagine a detective working on a case. He or she rounded up 10 suspects found near the crime scene and is now looking at them from behind a one-way mirror together with the only witness, who unfortunately didn’t see the criminal’s face. Now, in order to reduce the number of suspects, the detective will focus on asking questions about aspects the witness may remember, like height, hair-color, etc.
Equally, by asking you to label a well-chosen data point (which corresponds to the detective asking a new question), the machine can narrow down the possibilities for its parameters, hopefully coming closer to the “correct model”.
#3 Transfer Learning
Transfer learning is a brilliant way to train machines using a small amount of labeled data that is specific to your problem, together with a large amount of labeled data for a different but similar problem.
In an article published in the Cell journal in February of 2018, a group of researchers trained a machine to look at images of retinas (of a specific type) and tell whether they had one of a few “common treatable blinding retinal diseases,” which have to be diagnosed as early as possible.
Instead of starting from scratch, the researchers took their initial parameters from a neural network that was already trained on tens of millions of labeled data points from ImageNet, a database of images of all sorts of things, labeled with the names of the objects each image depicts. Then, they adapted the network to classify images into their own categories of interest (3 retinal diseases and 1 category for “normal/no disease”) and retrained it using only about 100 thousand labeled images of retinas. After 2 hours of training, the machine had already achieved a similar level of accuracy as the 6 experts to whom it was compared. To go even further and prove the usefulness of transfer learning for such applications, they took the original neural network (trained only on the ImageNet data points) and trained it again, but this time using only 1 thousand retinal images from each of their 4 categories. Training took only about 30 minutes and the results – obviously slightly worse – were still fairly close to the accuracy of the human experts and produced fewer false positives than one of them.
While it is unlikely that AI will be trusted to replace human doctors anytime soon, it could certainly become a helpful ally, for example to speed up the pre-screening process, eliminate obvious negatives, quickly recognize obvious positives, and so on. And because of the level of expertise needed to label data points in any area of medicine, this would be almost impossible to achieve without transfer learning.
These are just a few methods to make the most of advances in machine learning – to train a machine on a minimum of costly labelled data, and – with a limited budget – leverage that learning for your projects.
Please note: The opinions expressed in Industry Insights published by dotmagazine are the author’s own and do not reflect the view of the publisher, eco – Association of the Internet Industry.